Engaging the Data Science Community with Met Open Access API
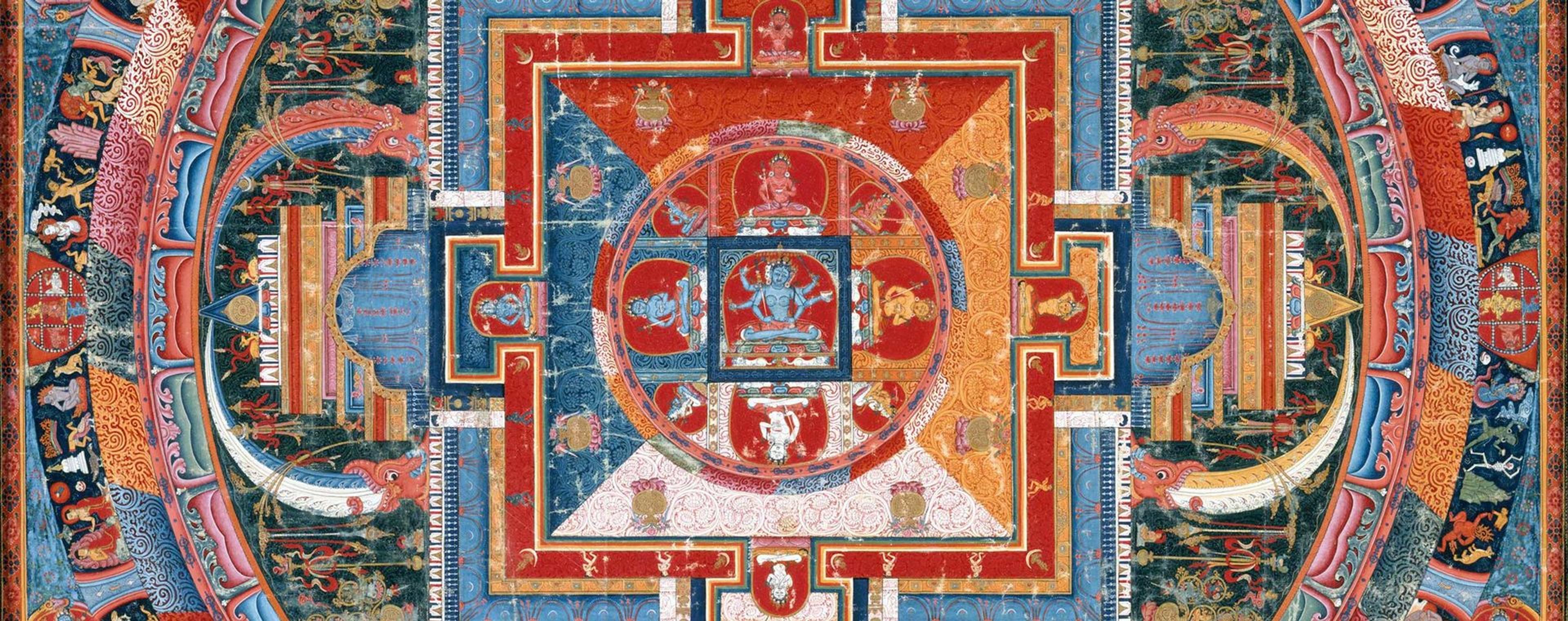
An image from the Met collection, packed objects which may be difficult for an algorithmic model to identify. Mandala of Jnanadakini, late 14th century. Tibet. Distemper on cloth, 29 1/2 x 33 in. (74.9 x 83.8 cm). The Metropolitan Museum of Art, New York, Purchase, Lita Annenberg Hazen Charitable Trust Gift, 1987 (1987.16)
Through the Museum’s Open Access program and the release of our public API, the Met collection is empowering its users in new and innovative ways. Among those who have worked with our data over the past year are members of the data science community. For example, our Open Access images are playing valuable roles in important data science research happening at Google, Cornell Tech, the University of Virginia, and the popular data science platform Kaggle. At these institutions, researchers in the field of computer vision– training computers to understand and recognize information in images–have been working with the Met collection to develop new algorithmic models for allowing their software to "see."
One particularly difficult challenge is teaching a computer model to properly tag artworks with keywords. Most models are trained to identify specific objects in images, like individual animals or street signs for self-driving cars. Breaking down what appears in artworks is inherently subjective, with no single correct answer: if a group of were asked to identify what is depicted in one of the Unicorn Tapestries, for example, they would likely come up with their own, unique answers . . . and all would most likely be right.
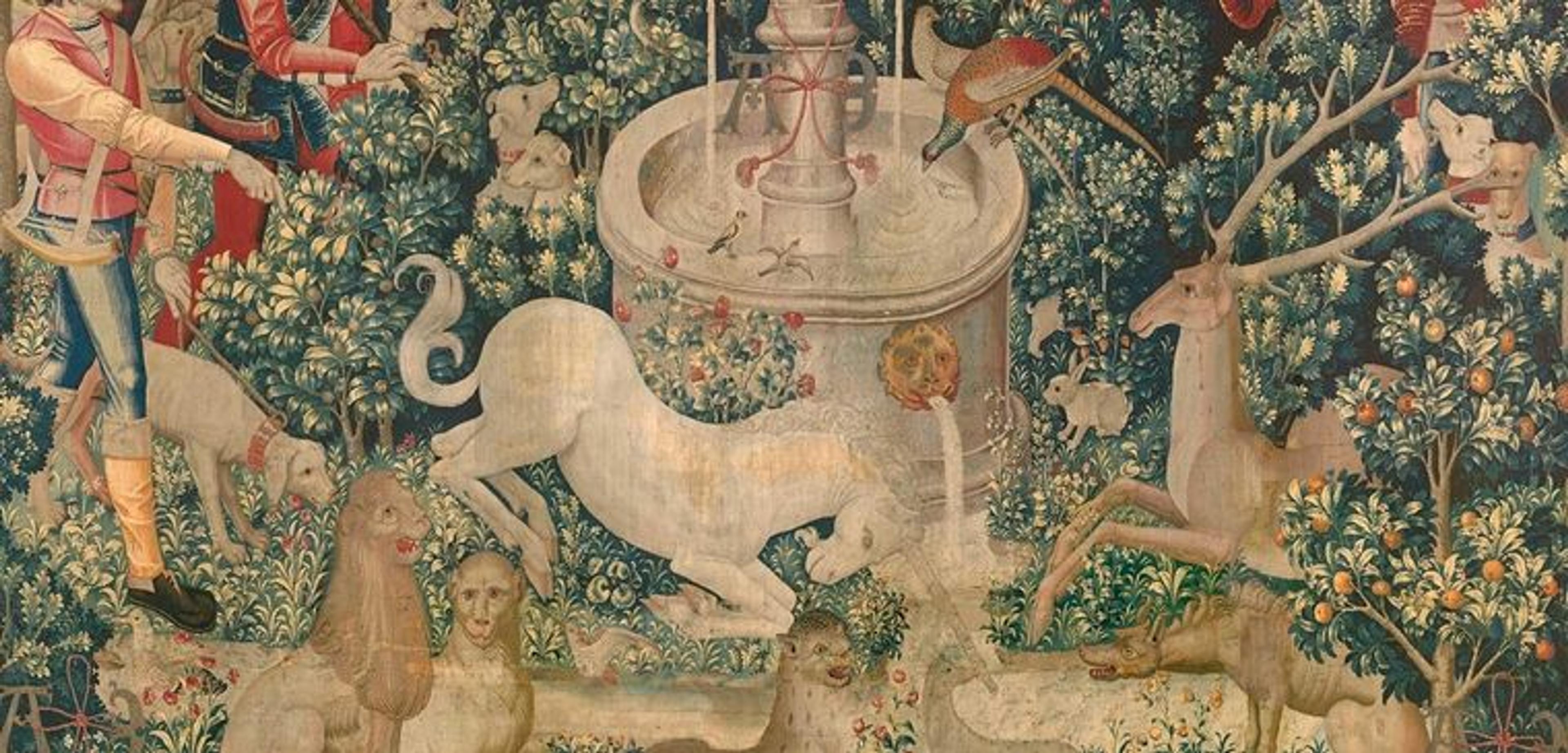
The Unicorn is Found (from the Unicorn Tapestries) (detail), 1495–1505. South Netherlandish. Wool warp with wool, silk, silver, and gilt wefts, 145 x 149 in. (368.3 x 378.5 cm). The Metropolitan Museum of Art, New York, Gift of John D. Rockefeller Jr., 1937 (37.80.2)
Complicating matters further, computer vision models also require an enormous set of training data: normally tens of thousands of records, if not more. We know from reviewing our human-created subject tags that 90% of the keywords we use at The Met are attached to fewer than 1000 objects. These numbers, which are comparatively low within the full scope of our massive collection, make it difficult for us to provide large amounts of training data. After all, we only have 600 artworks featuring cats!
Another challenge for data scientists is the diversity of the collection. The Met has nearly half a million artworks online, spanning more than 5,000 years of history, originating from locations and cultures across the globe. Figures from classical mythology, Buddhist deities, religious scenes, satirical material, animals, historical events, and human figures make up just a fraction of the subjects depicted in our collection. While it may be a simple task for human users, any machine learning model would need to identify these complex, nuanced subjects while working with only a small set of training data. Similarly, identifying gender has been a challenge for most machine learning models. Case in point: prior to the twentieth century, it was common for young boys to wear dresses. There are many objects in our collection that would be difficult for any computer model (or human) to identify without access to more contextual information, like seeing the word "boy" appear in the titles of the works.
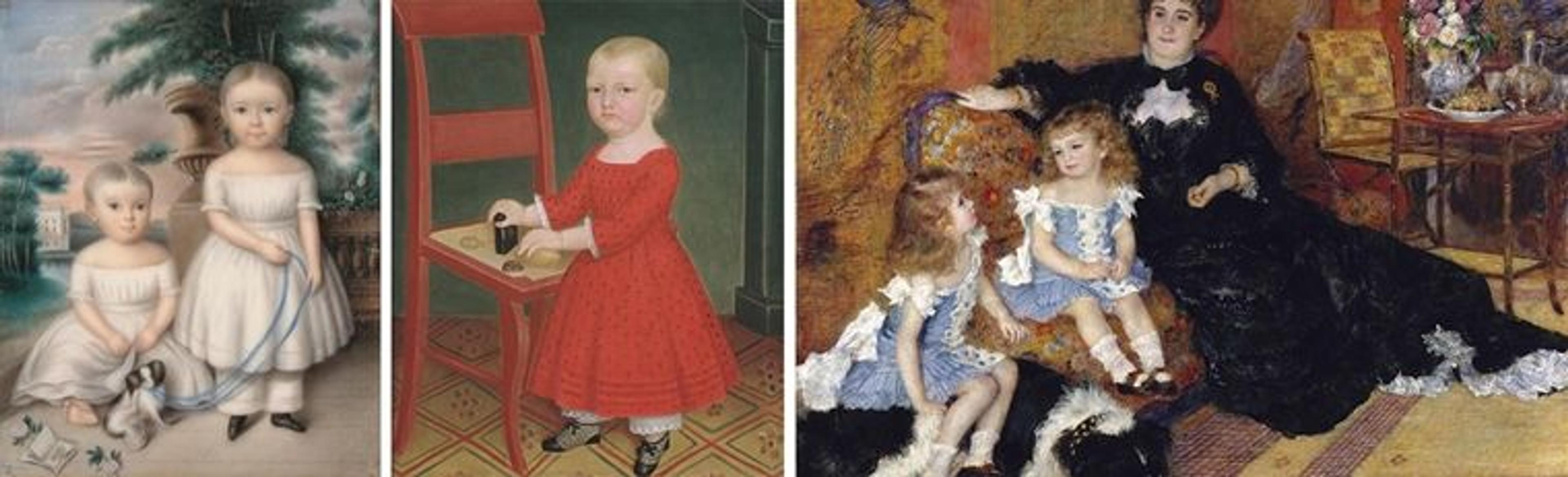
Left: Aramenta Dianthe Vail (America, 1820–1888). Watson van Benthuysen II and Thomas Van Benthuysen (detail), ca. 1837. Watercolor on Ivory, 4 5/8 x 3 11/16 in. (11.7 x 9.4 cm). The Metropolitan Museum of Art, New York, Dale T. Johnson Fund, 2005 (2005.175). Center: Boy with Blond Hair (detail), ca. 1840–50. American. Oil on canvas, 34 1/2 x 29 1/2 in. (87.6 x 75 cm). The Metropolitan Museum of Art, New York, Gift of Edgar Williams and Bernice Chrysler Garbisch, 1973 (1972.323.5). Right: Auguste Renoir (French, 1841–1919). Madame Georges Charpentier (Marguérite-Louise Lemonnier, 1848–1904) and Her Children, Georgette-Berthe (1872–1945) and Paul-Émile-Charles (1875–1895) (detail), 1878. Oil on canvas, 60 1/2 x 74 7/8 in. (153.7 x 190.2 cm). The Metropolitan Museum of Art, New York, Catherine Lorillard Wolfe Collection, Wolfe Fund, 1907 (07.122)
This past spring, our open access dataset was presented as part of a computer vision competition on Kaggle, a platform and online community focused on challenges related to AI and machine learning. We were excited to see the response from the data science community around our dataset. Our hope was that about twenty to thirty teams would compete. To our surprise, 521 teams from across the world participated over the course of the three-month competition. If you are interested in seeing how different models were created from our collection, you can view them here. Competition organizers also wrote a paper describing The Met's dataset, as well as the technical details about the challenge.
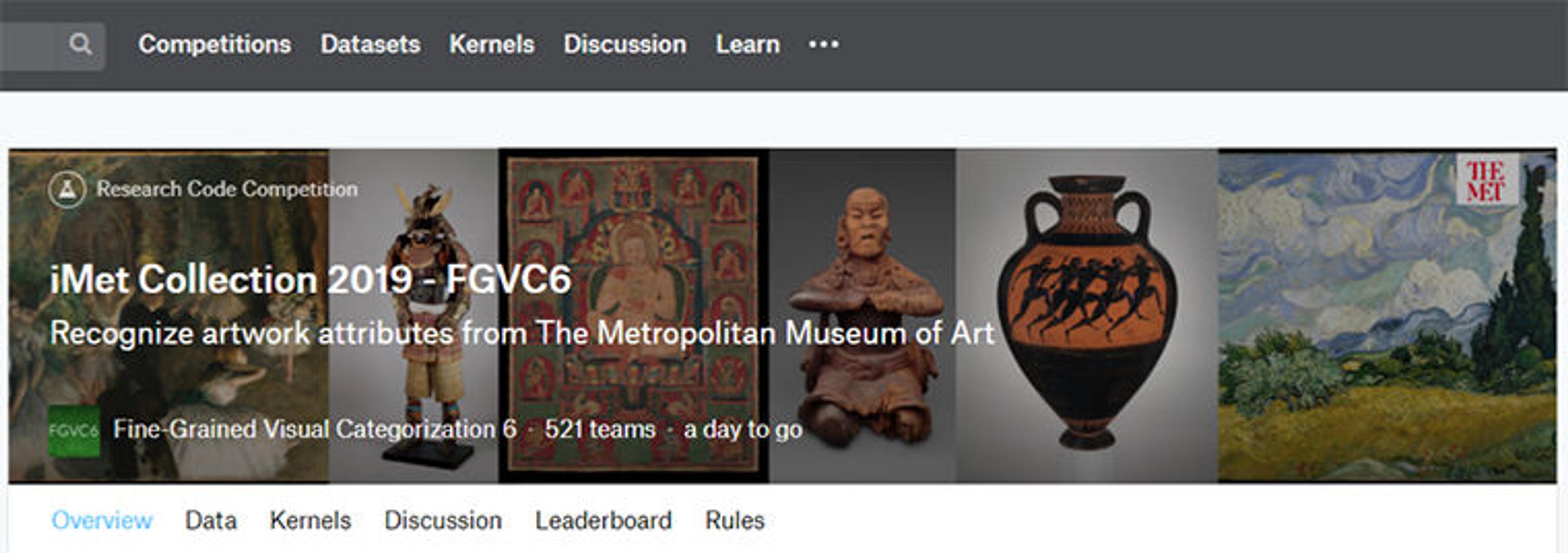
Kaggle, the AI and machine learning-focused platform and online community, held a computer vision competition to recognize artwork attributes using the Met Open Access API.
We are also working with students from the University of Virginia’s Data Science Capstone program, which tasks graduate students with solving real-world problems by collaborating with representatives from outside institutions. These students are working with our dataset to explore how machine learning can be used to identify subject matter in our artworks, as well as to assess bias in computer vision models. Working with the UVA students has been a great learning experience for us; we can’t wait to see the final results.
We still have a long way to go. While over 233,000 objects in our online collection have been updated with subject tags, we still have over 70,000 objects that have not and the process of tagging them manually is too arduous for us to undertake. While existing machine learning models do not produce tags at the level of accuracy or detail that we need, our involvement with the data science community has been incredibly rewarding, and a big step forward. We are seeing a new audience engage with and be inspired by the collection, and work advancing in a new scientific field that we hope will benefit other art collections around the world.
This is the second post in a three-part series celebrating the third anniversary of The Met's Open Access API. You can view the rest of this series here.
Jennie Choi
Jennie Choi is the general manager of collection information in the Digital Department.