Combining AI and Human Judgment to Build Knowledge about Art on a Global Scale
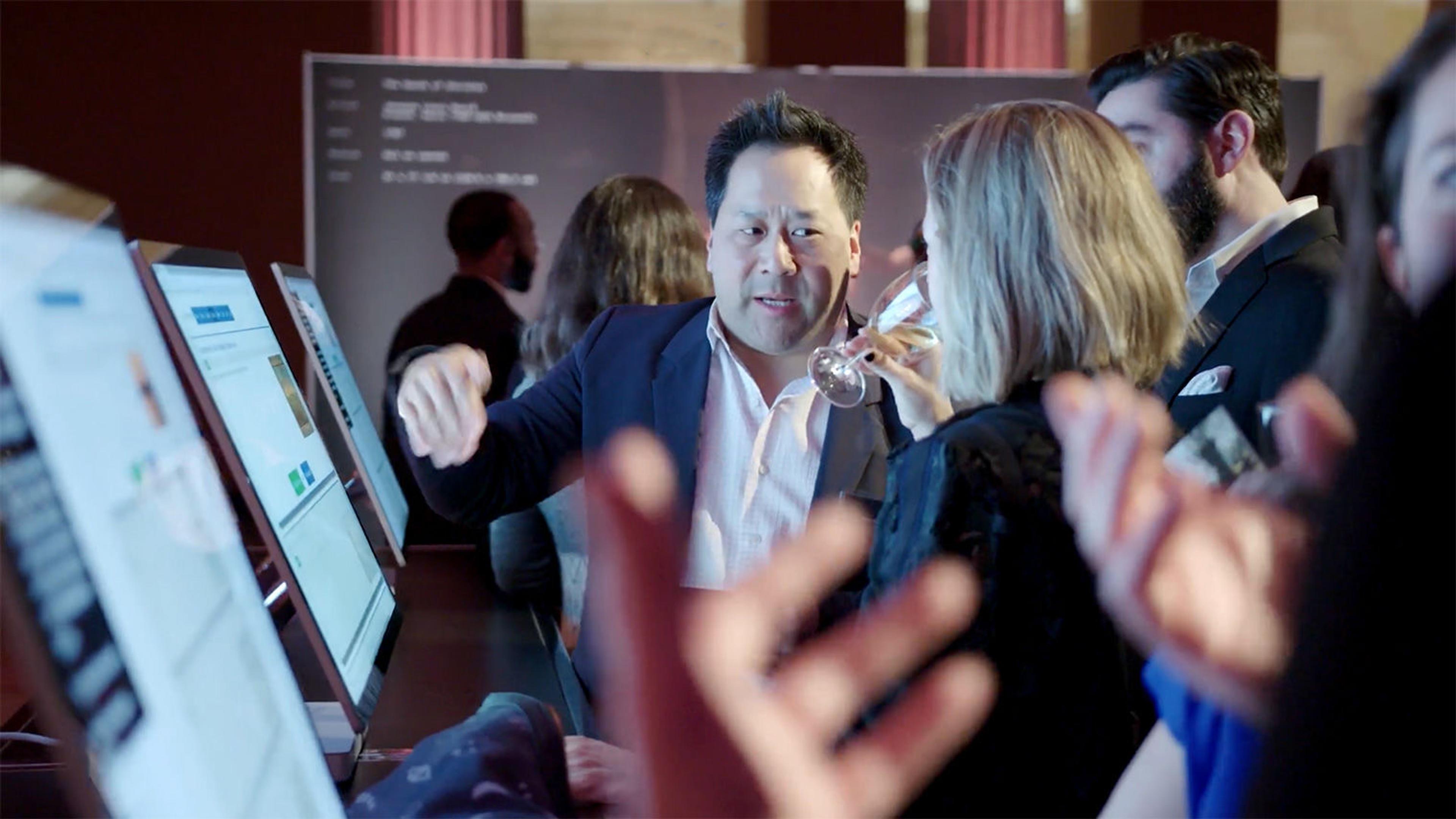
The author presenting Tag, That's It! at the reveal event in the Great Hall of the Met Fifth Avenue on February 4, 2019.
In early February, more than two hundred attendees, from pre-teens to leading art historians and technologists, gathered in The Met's Great Hall to classify artworks presented on digital screens. Using a simple game interface, participants tapped, dragged, and zoomed images of works in The Met collection to determine whether they depicted a house, boat, flower, or tree.
Though their gestures were simple, volunteers were actually doing something profound: they were assisting an artificial-intelligence (AI), or machine-learning, system in adding essential metadata to the works. With each tap and click, decisions about what an image depicts were collated to Wikidata (the free structured database of Wikipedia), which makes this descriptive metadata available to anyone in the world with access to the internet.
Twenty years ago, this kind of knowledge-gathering would have been considered the realm of science fiction, so you can imagine that night at The Met was a historic moment. Artificial intelligence is strong enough today, after years of development, to suggest usable characteristics, but the question remained: How could researchers mobilize good-faith humans to refine the raw results generated by the AI?
Over the past seventeen years, Wikipedia has demonstrated how humans can come together to gather knowledge, high-quality and at-scale. As the world's most visited reference site—published in hundreds of languages—Wikipedia is advancing new ways to access its volunteer expertise, such as through projects like Wikidata.
What's the Big Deal?
Simply put: Developing a standard structure for metadata in museums is a breakthrough for both scholarly and creative endeavors.
Museums typically maintain very descriptive metadata for their collections, including information like where objects are located, where the object was acquired from (its provenance), and, often, unique terms to describe an individual museum's collection. Since the institutions' needs for object preservation are generally prioritized higher than making metadata public, this information is not standardized across the cultural landscape. This presents a problem for technologists who are interested in studying objects across many museums.
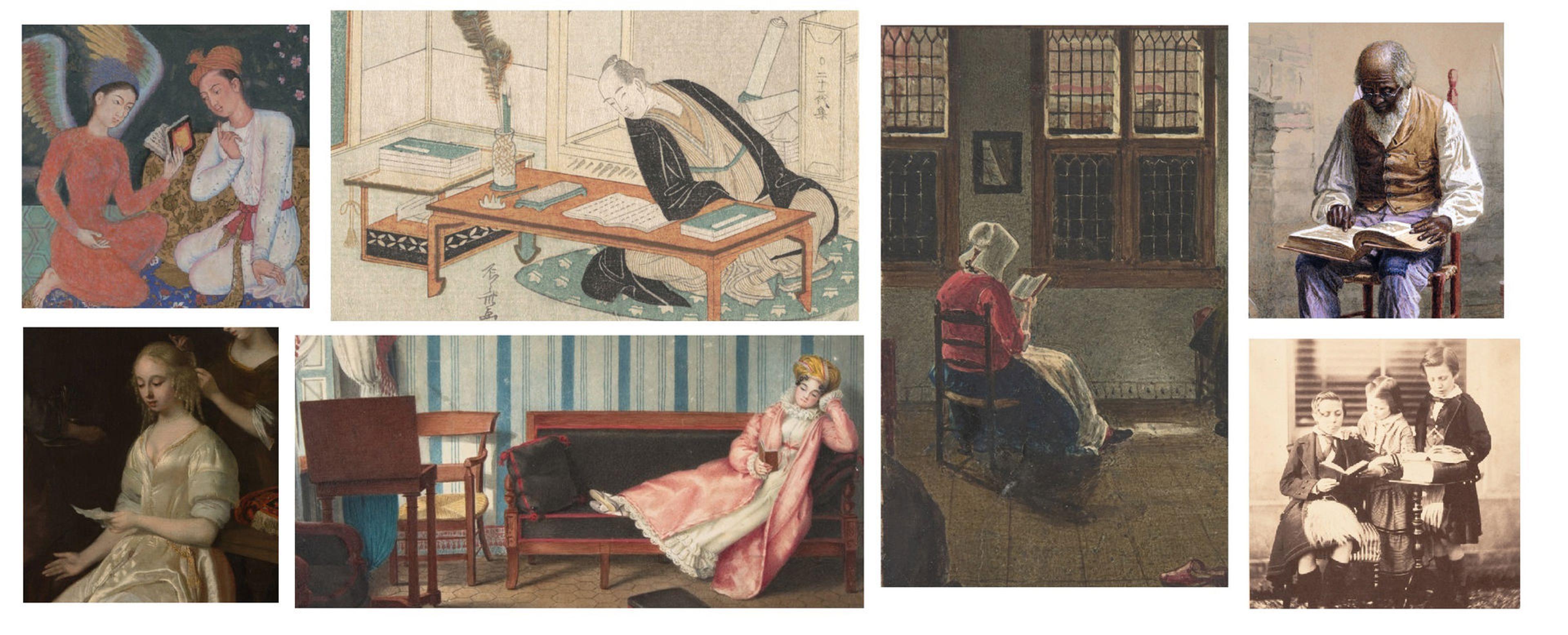
A selection of search results from The Met collection depicting the search keyword "reading".
In 2018, The Met began an ambitious subject-keyword tagging project for artworks in its collection. Chief Digital Officer Loic Tallon describes the project as the adding of "quality-controlled subject-keywords to the more than 300,000 digitized artworks in the collection." Of the 1,063 subject keywords, he gives examples ranging "from 'trees' to 'castles,' from 'floods' and 'portraits,' and from 'ritual objects' to 'cats.'" The goal of the initiative, Tallon notes, is to create consistent descriptive metadata that will be available to anyone, under a free license.
What kinds of possibilities might this tagging project create? Well, The Met, the Massachusetts Institute of Technology (MIT), and Microsoft held a hackathon at The Garage in Microsoft's New England Research and Development center in Cambridge, Massachusetts in mid-December 2018 to explore that question. In particular, participants were asked to investigate the use of artificial intelligence on The Met's new subject-keywords dataset. The Met invited me and other members of the Wikimedia community to experiment with more than 400,000 images, object records, and the new subject-keyword dataset, and to develop prototypes of AI-powered projects using The Met collection.
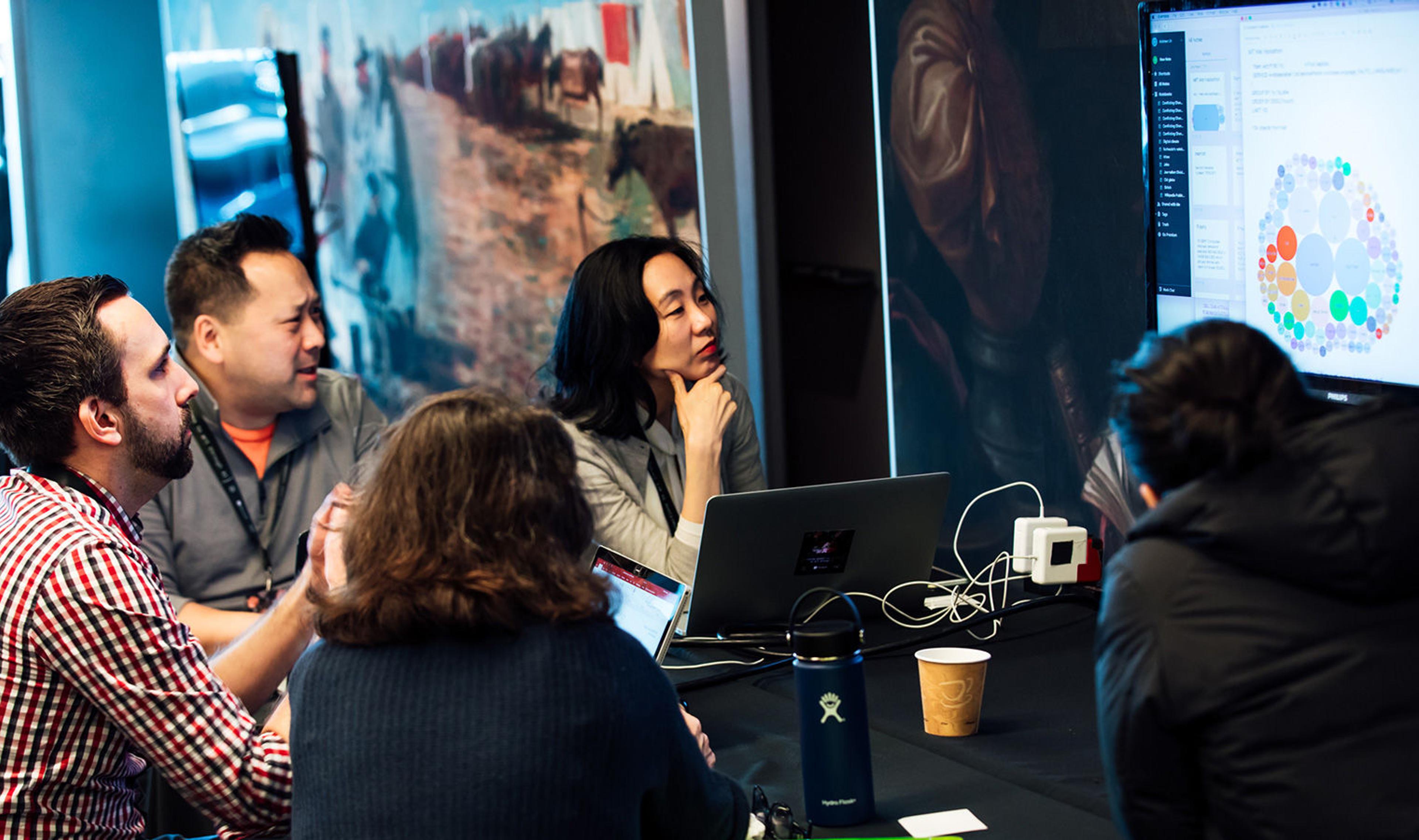
Participants working on Tag, That's It!, one of the AI prototypes developed at The Met x Microsoft x MIT hackathon in December, 2018.
In Cambridge, I worked with Jennie Choi, General Manager of Collection Information; Nina Diamond, Managing Editor and Producer (both in The Met's Digital Department); and Microsoft researchers Patrick Buehler, J. S. Tan, and Sam Kazemi Nafchi. Our team was inspired by The Met's tagging project to explore whether the keywords could be used to train a machine-learning model that could accurately predict tags for additional artworks. Using The Met's roughly 1,063 words of vocabulary and representative images to help train the model, we developed a proof of concept at the hackathon, which showed exciting results.
One of the challenges of using artificial-intelligence systems lies in how they handle substandard or partially accurate results—for example, an AI might guess boat when the answer is actually boulder. A system trained with a particular set of images and a fixed vocabulary of terms will produce a list of predictions with different levels of confidence, ranging from zero to one hundred. In just the last few years, image-recognition technology has taken a leap forward, with competition among companies and academics greatly advancing the state of the art. Systems that achieve 80 to 90% accuracy in many applications are increasingly common. But even such a high measure of confidence becomes useless if one cannot sift the incorrect classifications from the correct ones. This is where the Wikimedia community comes in. Its global network of skilled volunteers, we knew, would add that crucial element needed to solve this problem: human judgment.
Working with the Wikidata Game
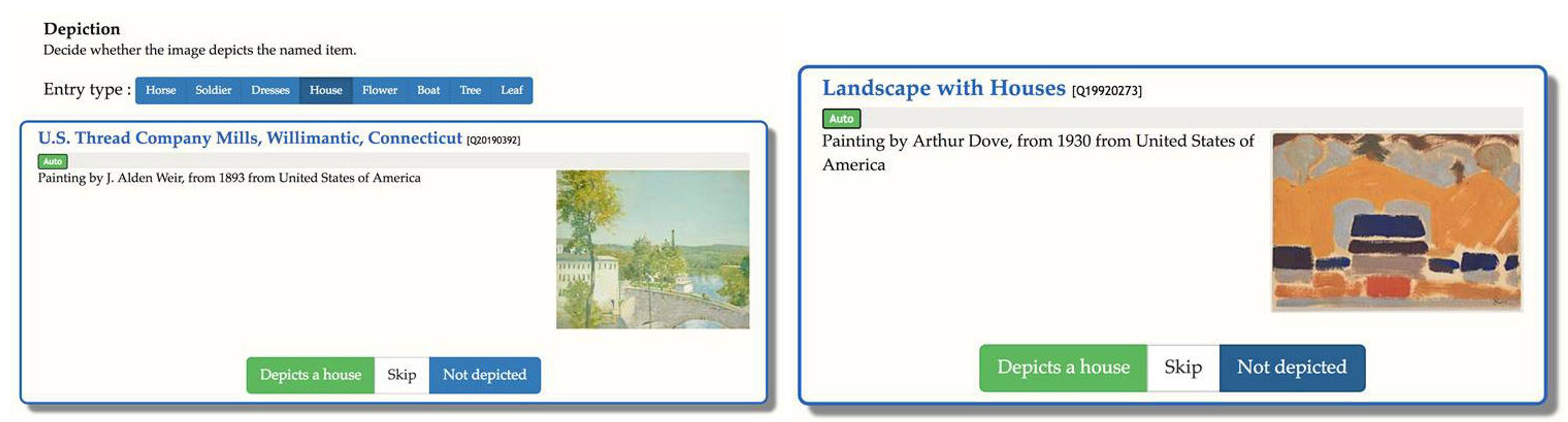
Screenshots of Depiction on the Wikidata Distributed Game system.
So we connected The Met's subject keywords to Wikidata and processed images that the model had never seen before. We achieved impressive results. Features from landscape-style paintings, such as trees, mountains, and animals, were recognized with excellent accuracy. Our next step was to create a way to reliably accept or reject these AI-generated suggestions. Fortunately, Wikidata already has a game interface we could utilize to have people help with repetitive decision-making tasks. When presented with a simple choice, Wikidata volunteers simply press a button: yes, no, or skip. Every day, hundreds of volunteers use the Wikdata Distributed Game system, and together they make thousands of decisions on numerous tasks, such as adding new database items or removing redundant information.
After the hackathon ended, we wanted to continue developing our prototype, so I worked with The Met to map their specific vocabulary to Wikidata items. Such a map is called a "crosswalk database," and it has multitudes of value. For example, since Wikidata is a "semantic" database that models concepts, a search for "mammal" in Wikidata can return artworks labeled with "dog" or "horse." Traditional, exact-match, lexical searches, such as The Met's, cannot. This also means that searches on Wikidata can be conducted in any language. An English speaker searching for "dog" would get the same results as a Spanish speaker searching for "perro" or a Japanese speaker searching for "犬." This reason alone has been a motivating factor for many cultural institutions to integrate with Wikidata.
On January 31 we launched a program on the Distributed Game system called Depiction, which was loaded with the keyword suggestions generated by our AI. After successful testing, we revealed the Wikidata game to the public at the February 4 event in the Great Hall, where we recorded more than seven hundred judgments via button presses by participants—a powerful demonstration of how to combine AI-generated recommendations and human verification. Now, with more than 3,500 judgments recorded to date, the Wikidata game continues to suggest labels for artworks from The Met and other museums that have made their metadata available.
One benefit of interlinking metadata across institutions is that scholars and the public gain new ways to browse and interact with humanity's artistic and cultural objects. Wikidata's query function, for example, provides powerful tools that can be used to pinpoint trends in painting or the prevalence of women artists over time. Other tools, like Crotos (a search and display engine for visual artworks) and Wikidata Timeline allow anyone to browse visual collections in one place. With Wikidata, human knowledge and creativity are modular, structured, and explorable in ways we are only starting to discover.
Learn More
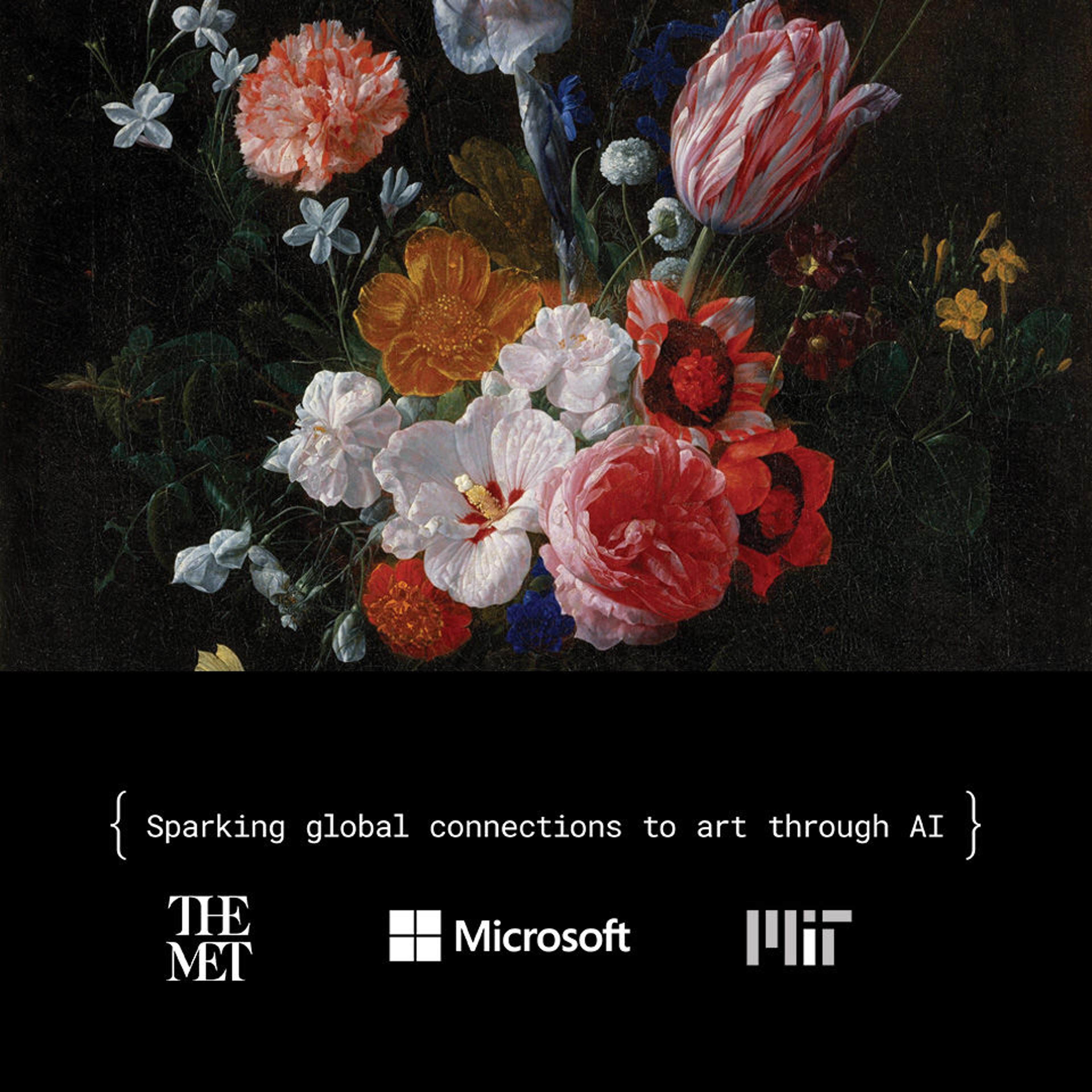
The Met, Microsoft, and MIT held a two-day hackathon session to explore how artificial intelligence could connect people to art. Learn about this collaboration and the prototypes it generated.
Learn more about The Met's Open Access initiative.
The Met's Open Access initiative is made possible through the continued support of Bloomberg Philanthropies.
Andrew Lih
Andrew Lih is an open knowledge and digital strategist and principal investigator of the "Wiki Art Depiction Explorer," a Knight Foundation-funded project in conjunction with the Smithsonian Institution and Wikimedia District of Columbia. He is the author of, The Wikipedia Revolution: How a Bunch of Nobodies Created the World's Greatest Encyclopedia.