Picture, if you can, the history of art. What does it look like? You can probably call up certain images and ideas—specific paintings and sculptures, the sounds of music and dance—or perhaps you know the characteristics of what we call Cubism, or the traditions of shan shui painting. But zoom out further still. How do you visualize the structure underlying artistic creation? One model is, of course, the modern museum. But there is another model—made possible by artificial intelligence—that may provide an outlook difficult to translate in brick-and-mortar institutions.
An idea central to an encyclopedic institution like The Met is that there is no single “art.” There are about two million works of art in The Met’s collection, spanning thousands of years, and no promises are made that you can understand the entire project if you just stand right over here and look at it from such-and-such angle. But there is, implicit in the Museum, an understanding that probably every culture across the world has made images, and it can therefore be fruitful to ask: Why?
“One handicap of museums is that we deprive certain objects of their cultural context with the very act of displaying them in a gallery,” Kim Benzel, curator in charge of the Department of Ancient Near Eastern Art, said. In many ways, her department encourages visitors to move beyond ideas about art and authorship that are influenced by European workshops and markets, and instead understand culture as a “liminal, not literal” space. Even cuneiform must be understood as operating within a larger system, rather than as something more closely approximating modern written language.
Benzel recently attended a two-day hackathon hosted by The Met, Microsoft, and the Massachusetts Institute of Technology (MIT), in which participants gathered to explore how artificial intelligence can connect people with art. There, she met contemporary artist Matthew Ritchie, computational neuroscientist Sarah Schwettmann, and Microsoft software engineers Mark Hamilton and Chris Hoder. Benzel quickly discovered they shared similar sensibilities about art and authorship.
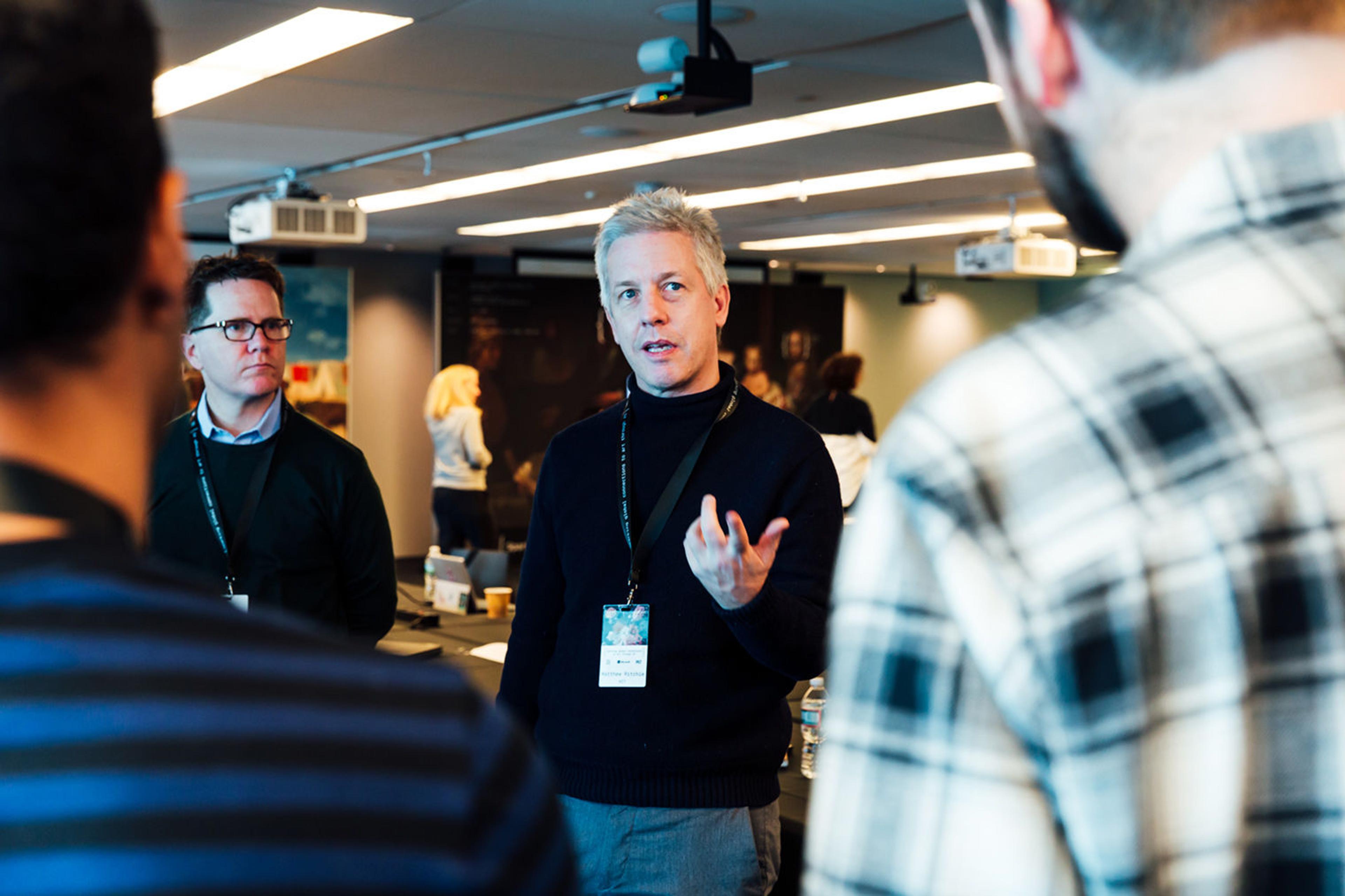
Matthew Ritchie, Dasha Zhukova Distinguished Visiting Artist, MIT Center for Art, Science, and Technology. Photo © Natasha Moustache/Listen
“I immediately got to understand that, ironically, what scares people about AI is exactly what makes the Ancient Near East difficult for visitors: they’re both perceived as inaccessible because they live in these in-between spaces, these dreamscapes where we don’t let ourselves go,” Benzel said.
Many of the objects in her department’s collection, which date as far back as the eighth millennium B.C., were created within cultures who held very different understandings about art and authorship than many of us do today. As an example, Benzel pointed out that many languages don’t even have a word for “art,” and that the objects we see in the Ancient Near Eastern galleries were often ritualistic or utilitarian in purpose. Many of them were never meant to be seen; others were meant to be deliberately destroyed after their ritual use expired.
“Ancient Near Eastern images are not mimesis—when you give image to something, in most of the Near East, they’re as good as the biological or physical thing that it represents. By giving image to something, you’re giving it life,” Benzel said. And none of this, importantly, really has much to do with the artist’s individual identity.
This conception of mutable culture can be hard to grasp in galleries where fragments that shouldn’t have even survived are stored safely under glass bonnets; cuneiform texts evoking gods were originally buried underground but are now mounted in vitrines; and objects that were destroyed because their power was too great to be left unattended are reconstructed in scientific labs. But perhaps there is a way that technology can help us better comprehend this missing dimension or regain access to this imaginative and powerful realm.
Ritchie, who is the Dasha Zhukova Distinguished Visiting Artist at the MIT Center for Art, Science, and Technology, agreed. Machine learning, he said, makes it possible to begin visualizing the diversity and complexity of artistic creation. At the event, hosted at Microsoft’s New England Research and Development Center in Cambridge, Massachusetts, Benzel, Ritchie, and Schwettmann worked with artists, scholars, and technologists spread across the three organizations to begin exploring how artificial intelligence can model what Schwettmann referred to as the “latent space underlying types of creation that you see in The Met.”
One of the tools that was developed, using Microsoft’s artificial-intelligence platform, analyzed The Met’s collection of ewers (a kind of jug). Schwettmann, Ritchie, Hoder, and Hamilton have since expanded this into a larger prototype called Generist Maps that will be showcased during a private event in the Museum’s Great Hall on February 4. Their premise, as Schwettmann and Ritchie described it, is fairly straightforward: All of the objects in all of the museums across the world represent only a fraction of the art that has ever been created.
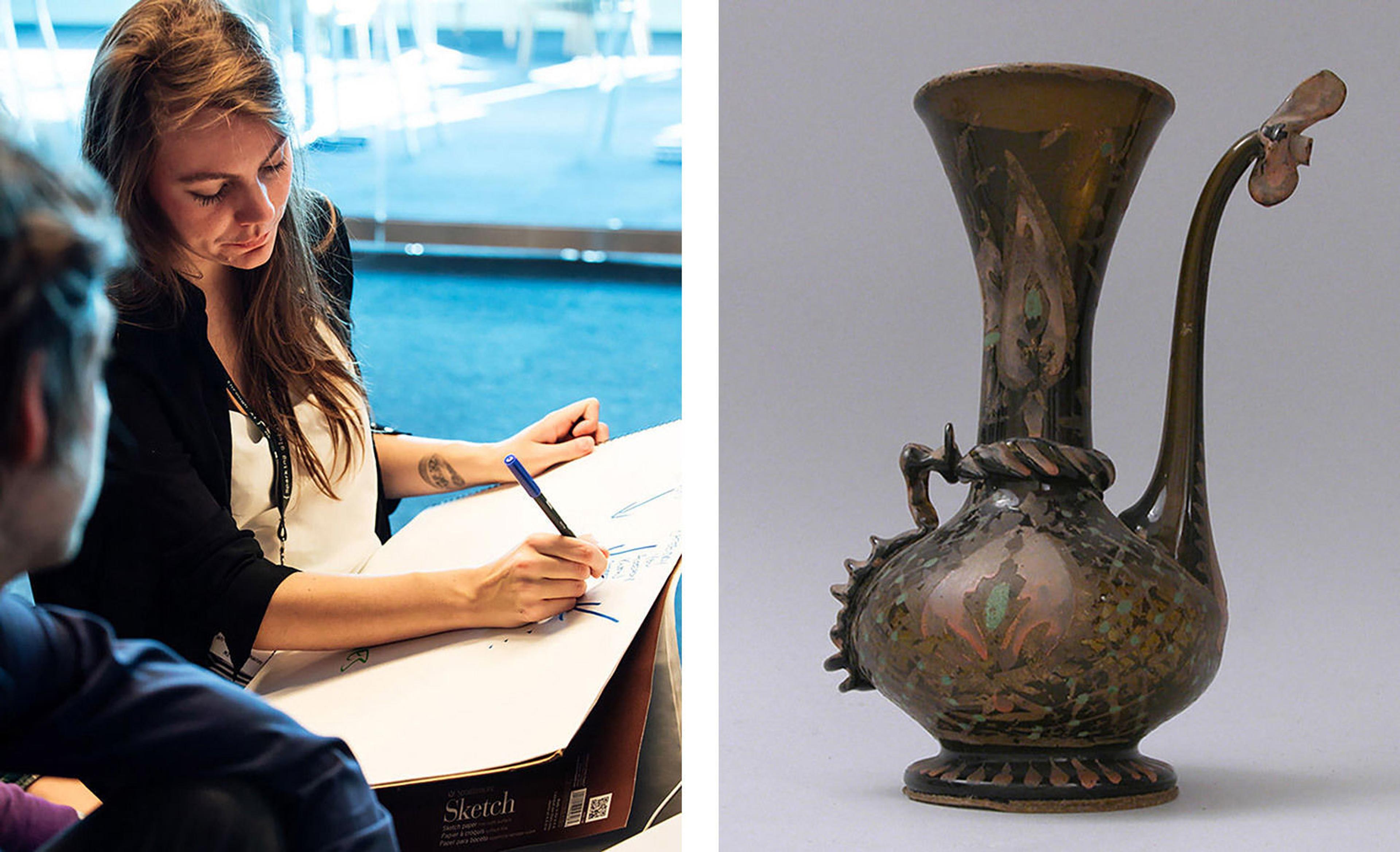
Left: Sarah Schwettmann, Computational Neuroscientist, MIT. Photo © Natasha Moustache/Listen. Right: Ewer, probably 18th–19th century. Attributed to Iran. Painted glass, 7 ½ × 5 ½ in. (18.7 × 14 cm). The Metropolitan Museum of Art, New York, Edward C. Moore Collection, Bequest of Edward C. Moore, 1891 (91.1.2062)
Those at The Met have been preserved because of their cultural import, but even the most extensive museum collection is inherently unable to give the total picture of the history of art. Inevitably, individual objects begin to stand as metonyms for much larger concepts, and nuances like the ritualistic aspect of a particular work, for example, can be at risk of being flattened out when displayed statically in the galleries. But by harnessing artificial intelligence, it’s possible to envision artworks that may have existed, based on our knowledge of artworks that we know to exist, and in that way gain a fuller, more complete understanding of visual culture.
“Our project offers an opportunity for the entire world to experience not just the objects, which have a tendency to collapse you into thinking, ‘Oh, that’s the best bone saddle I’ve ever seen,’” Ritchie said. “This reopens that discourse and reminds you that art is a mutable space.”
Generist Maps makes use of technology called a generative adversarial network (or GAN), which is a type of neural network. Essentially, a GAN is composed of two parts: The first, called a “generator,” produces images based on what it knows about that category of image. Tell it to make a landscape, for example, and it figures there’s probably an earthy palate, perhaps some trees over here, and so on. The second part of a GAN is called a “discriminator,” and this half of the network checks the other half’s work: it might determine picture A doesn’t look enough like a landscape, but picture B does, so let’s toss A, and B can stay. What a GAN ends up making is a new image that ostensibly fits within the category for that kind of image.

In this simulation, the GAN underlying Generist Maps visualizes the “latent space” between different kinds of objects in The Met’s collection. Image courtesy Sarah Schwettmann
The use of GANs is not uncommon in many contemporary artists’ practices, where they’re often employed as tools for generating images, not unlike a camera or Photoshop. Hamilton said, “In the past, this technique struggled to generate realistic-looking images. The secret to algorithmic art lies in starting simple, and slowly showing the algorithm more complex art over weeks of training.”
What makes this prototype particularly compelling is its dataset: The Met’s online collection. This February marks the second anniversary of The Met’s Open Access program, which makes hundreds of thousands of works of art available online. Ritchie and Schwettmann noted that a curated collection—particularly one which extends millennia—provides a different lens toward what constitutes human cultural production, as opposed to a crowd-sourced collection such as Wikipedia, which is weighted by popular artists and art forms, like European paintings. In Generist Maps’s case, the GAN powering the prototype looks at the areas of art represented within The Met’s collection and infers the “latent space” between them by simulating intermediary images.

In this simulation, the GAN underlying Generist Maps visualizes the “latent space” between ewers and vases in The Met’s collection. Image courtesy Sarah Schwettmann
“This model is an extrapolation of curation, by virtue of what made it into a collection,” Schwettmann said. “These kinds of machine-learning models can internalize the features selected for by contemporary curation—as well as the historical evolution of collectively, iteratively produced cultural artifacts—and suggest what the rest of that space could look like.”
Schwettmann likened Generist Maps to a topographical map. “Marked” on that map are actual artworks, sourced from The Met’s online collection. The Museum has tagged these images with identifiable characteristics, which Generist Maps uses to suggest possible instances of artworks that fit somewhere between actual existing artworks. By plotting out these objects, the GAN is able to infer possible variations of objects as they may have existed, given what we know about how and when they do exist—that is, it’s imagining a third thing between two known things. Do this once and you get a recombinant image that can be fun to tinker with and contemplate, but do it enough times and eventually a larger cohesive picture starts to emerge.
Ritchie and Schwettmann were quick to stress that their group has not developed “an art-making machine.” Nor is Generist Maps an attempt to extrapolate an omniscient or monumental view of the entire history of art. “I don’t think anyone’s trying to make any claims that we’ve solved where art is, or that we’ve got art in a box here,” Ritchie said. They also acknowledge that artistic decisions made by model designers have ethical implications that cannot be ignored, such as the degree to which a GAN takes context and provenance into account.
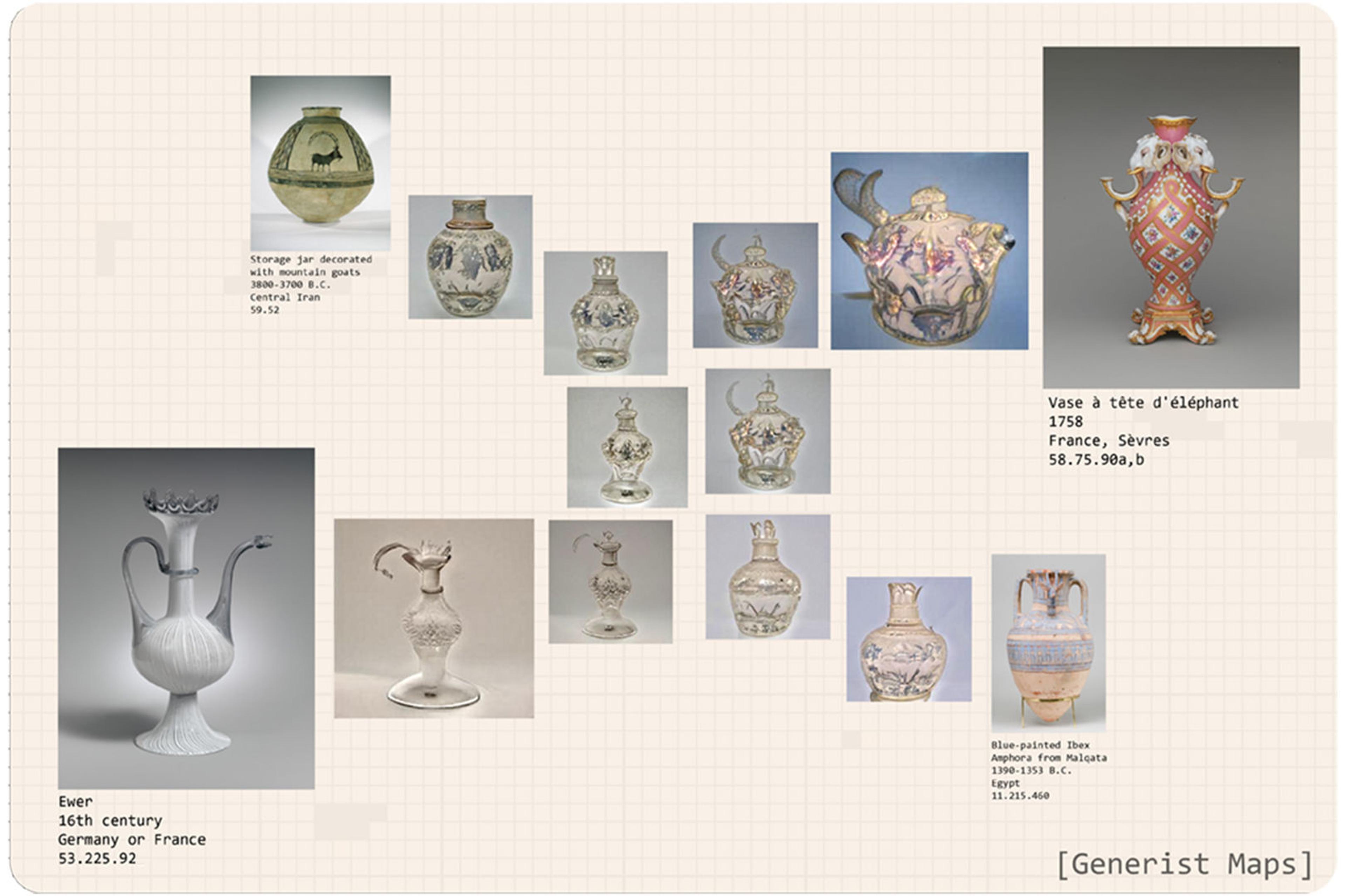
This diagram illustrates the “topographic map” generated by Generist Maps. At each of the four corners are vases or ewers in The Met’s collection. The ten objects in between are inferred from the latent space by the GAN. Image courtesy Sarah Schwettmann
What it is, instead, is just the beginning of a deeper look at the history of art and cultural production—one enabled by machine learning and artificial intelligence. Beyond that, Ritchie hopes that Generist Maps can be understood as part of the long histories of systematized aesthetics and artistic imagination:
“Think of Max Ernst. He’s a classic example of recombinant work. He was taking stuff out of encyclopedias, which were the dominant knowledge form of the day. All his books are from one set of the Encyclopedia Pedagogica, which he was then recombining into this dreamscape where knowledge is destabilized. No one thinks about Max Ernst and goes, ‘Oh, he ended art because he recombined things.’ You know, ‘That was it!’ In fact, what you’re doing is kicking off a whole new realm of aesthetic exploration.”
Schwettmann took this metaphor for Generist Maps one step further, stating, “It’s iterative Surrealism.”
And as for Benzel? She’s watching the prototype develop, with interest. She thinks machine leaning has the potential to help The Met’s visitors navigate and understand its collection from a point of view that is more aligned with ancient and non-Western conceptions of art and image-making. She sees possibility in how artificial intelligence can challenge or reconfigure entrenched notions, and accommodate multiple points of view. “What happens when you start un-editing and letting more people in?” she asked. “Can that be a way to start understanding shared experience?”
Marquee: Members of a hack group exploring connections between art and artificial intelligence were influenced by Assyrian reliefs and imagery of a “sacred tree.” Relief panel (detail). Gypsum alabaster, 90 ½ × 84 ½ × 6 in. (229.9 × 214.6 × 15.2 cm). Mesopotamia, Nimrud (ancient Kalhu). Neo-Assyrian, ca. 883–859 B.C. The Metropolitan Museum of Art, New York, Gift of John D. Rockefeller Jr., 1932 (32.143.3)